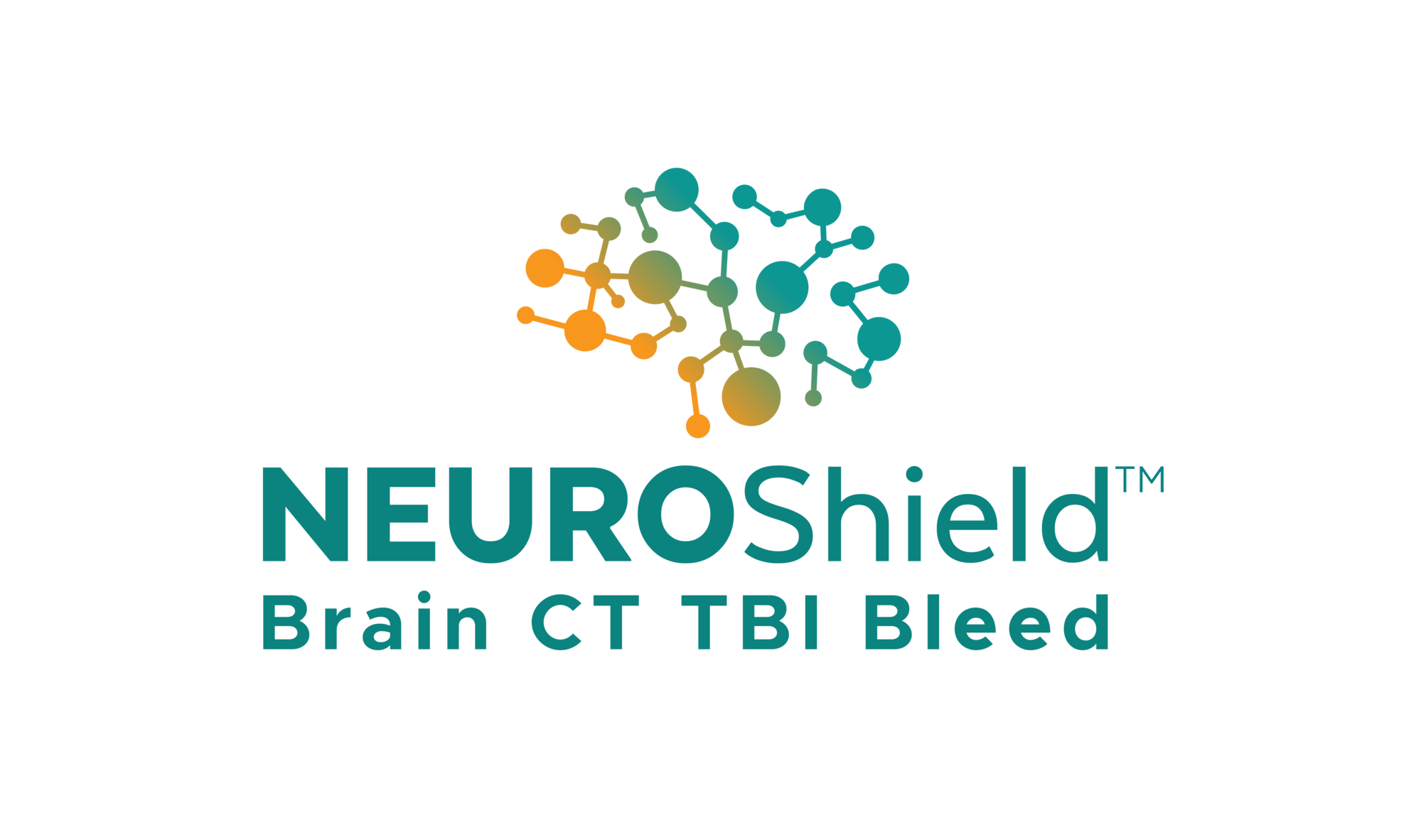
Decipher the Silent Epidemic
NeuroShield CT is an AI enabled Tool to optimize the “Golden hour”in neurotrauma.
Neuroshield CT automatically screens all the Head CTs in a hospital system, identifies cases with pathologies such as bleeds, fractures, midline shift and flags them to the radiologists and clinician team thus helping them manage their workflow more efficiently by prioritizing cases that need the golden hour intervention on a timely basis.
Neuroshield CT also provides a detailed analysis report. This quantifies and locates the bleeds, fractures and midline shift thus providing most valuable information on a timely basis that will help the acute care team triage effectively and devise optimal treatment plans for the patients in their hour of need.
The product is integrated into the clinical and radiology workflow and efficiently prioritizing critical cases that require immediate attention; reduces radiologist burnout and overload.
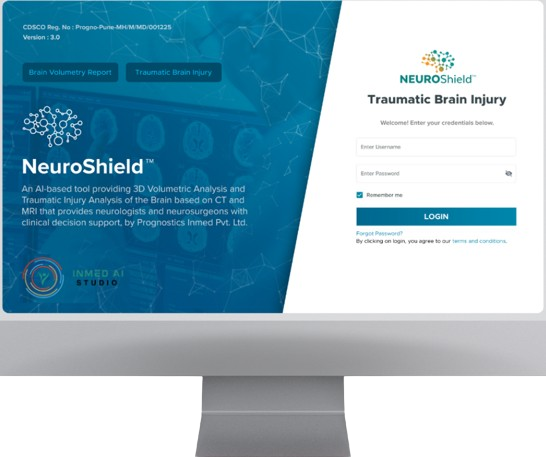
Empowering Possibilities: What We Can Do for You
Benefits
- Hyper fast.
- Accurate quantification.
- Helps manage acute triage in the case of neurotrauma.
- Saves radiologist time and reduces radiologist burnout.
- Prioritize cases even when radiologist is not available.
- Realtime communication.
- Easily and fully integrated.
- Cloud native and on premise version deployment.
What Experts Say
How We Do It?
WE RESEARCH
InMed distinguishes itself by prioritizing extensive research, paving the way for groundbreaking advancements in
A 2D U-NET combined model based on lesion size for automated stroke lesion segmentation
The 2D U-NET architecture used in segmenting the ROIs is based on the format to include the lesion size, lesion volume and their locations in the left and right hemisphere of the brain, and cortical and subcortical brain regions. The dice score for this model was 0.822 on the testing set. This result shows that considering the lesion sizes and volumes can help in obtaining a precise and automated segmentation model.
Automated Midline Shift Detectionand Quantification in Traumatic Brain Injury: A Comprehensive Review
This review summarizes the current state of research on AI-based approaches for MLS analysis in TBI cases, identify the methodologies employed, evaluate the performance of the algorithms, and draw conclusions regarding their potential clinical applicability.The findings highlight the importance of AI techniques in improving MLS diagnosis and guiding clinical decision-making in TBI management.
Automated,intracranial hemorrhage detection in traumatic brain injury using 3D CNN
We developed a 3D CNN model for automatically detecting the ICH from head CT scans. The screening tool was tested in 20 cases and trained on 200 head CT scans, with 99 normal head CT and 101 CT scans with some type of ICH. The final model performed with 90% sensitivity, 70% specificity, and 80% accuracy.
Automated detection of intracranial hemorrhage from head CT scans applying deep learning techniques in traumatic braininjuries:A comparative review
3D CNN-based Automated Detection of Midline Shift in Traumatic Brain Injury Cases from Head CT scans